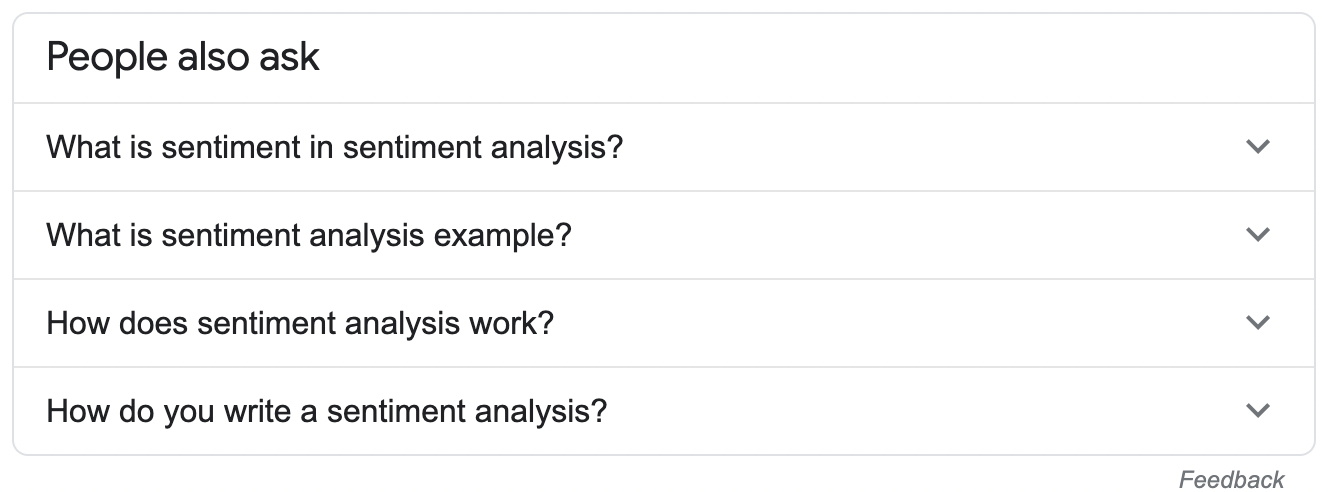
11 Feb What is Sentiment Analysis? – AI in Social Media
What is Sentiment Analysis? – AI in Social Media
Not so long ago, AI seemed like a concept straight out of a science fiction movie. Nowadays, we see it virtually everywhere. Manufacturing, healthcare, finance, transportation, social media—all of these industries are based on AI to a great extent. Another sector which benefits from AI and machine learning is marketing. AI has a hand in a massive amount of our marketing activity from putting keyword clusters together and utilising chatbots to personalisation and text analysis.
One of such uses of AI and machine learning is sentiment analysis. Innovative marketers use this to their advantage to monitor brand reputation, gather feedback and avoid PR crises. Here’s how sentiment analysis works, including the different types, the benefits, and the best tools.
Defining sentiment analysis
Put merely; sentiment analysis is the practice of defining whether a statement has a negative, positive, or neutral sentiment. More advanced sentiment analysis tools have the power to establish whether the message is emotionally sad, happy, or angry.
AI and machine learning make sentiment analysis possible, as the algorithms can learn by zooming through tons of data. The more data an algorithm analyses, the more accurate the result. To acquire the required information, you need software to conduct social listening. Generally speaking, this is the act of gathering all of the mentions of a particular keyword online.
“In 2021, competition is fierce and, as such, it’s never been more important to keep an eye on what’s being said about your brand and your offering. I use sentiment analysis tools constantly to make sure there’s nothing negative being said about my brand and to nip any issues in the bud,” says Jacek Żmudziński, senior associate at Future Processing
Such monitoring is not only limited to social media. Tools can analyse the mentions containing a specific keyword from all over the internet.
Because all of the views and opinions are given by people are subjective, we can assign a sentiment to them. Based on certain words, we can identify the author’s feeling towards something. Establishing whether a statement was subjective or objective is not that simple. Let me illustrate:
All bachelors are unmarried. -> this sentence is factual, and it is objective.
All unmarried people are lonely. -> this is an opinion, a subjective statement.
Distinguishing between these becomes an entire problem of its own. Subjective statements are based upon one’s feelings, predictions, and experiences—the algorithm needs to know that.
A metric called sentiment score has been established by sentiment analysis professionals to help assess opinions. Sentiment score is pretty straightforward to calculate—it consists of sweeping statements, total negative statements and absolute positive statements. Still, different tools use different methods and algorithms of recognising the positivity and negativity so that the sentiment score may differ from app to app.
Example of a mention with a negative sentiment
“These days, there’s a huge amount of chatter on the internet, and it can be tricky to keep up with what’s being said. Sentiment analysis is hugely important for us, and we use it to extract comments for use as testimonials and look up the sentiment for Krakow. These days, any business who isn’t using these tools are opening themselves up to potential problems as well as missing out on opportunities.” says Jacek Ptak, CEO & Founder of KrakowDirect
Behind the scenes of sentiment analysis
Sentiment analysis is quite a complicated process—it is based on Natural Language Processing (NLP), computational linguistics, and text analysis. Because language is such a complex communication medium, there’s a lot to analyse. The study of meaning—semantics—and the rules of a particular language—syntax—are the two schools which help immensely with analytics.
Of course, sentiment analysis is not entirely perfect. This can be evaluated manually by checking which judgements made by the algorithm correspond with our human perception. Still, sentiment analysis tools can recognise the feelings correctly about 85% of the time.
There are three primary types of sentiment analysis:
- Fine-grained
This method involves the classification of sentiment on a five-point scale. These levels are: very negative, negative, neutral, positive, and very positive. Since you don’t only base this on your regular two-way polarity, the fine-grained analysis provides much more accurate results.
- Emotion AI
Because there are countless emotions, it becomes increasingly difficult to distinguish between the more subtle ones. Still, sentiment analysis for emotions exists, and it’s able to perceive feelings such as sadness, anger, happiness, frustration and boredom. This is especially useful for reputation management and brand monitoring.
- Aspect-based
Different products have different features, and these features can be described in various ways. For example, a review statement stating that the screen is too small is negative, although it doesn’t contain any negatively-perceived words. With aspect-based analysis, it’s within reach to capture subtleties in opinions about products or services.
There’s also a different division of sentiment analysis, which focuses on the methods:
- Knowledge-based
This method is based on the presence of particular words and the available resources and lexicons. By detecting feeling-containing phrases, a knowledge-based approach of sentiment analysis can assign an emotion to the statement. While it’s easy for unambiguous terms such as sad, happy, or afraid, it becomes increasingly challenging when the algorithm is presented with non-obvious expressions.
- Machine learning
Machine learning methods are instrumental when analysing large amounts of text. Because the amount of data that machine learning methods can inspect is so huge, it’s possible to identify the person behind a statement. This, in turn, makes associating certain products and reviews with a specific type of person easy.
Hybrid systems involve combining the previous two methods. They’re instrumental at recognising subtle changes in emotion. Hybrid approaches are based upon semantic networks, ontologies and machine learning algorithms.
“Sentiment analysis has been essential for my brand in helping to see how people feel about it. Just as importantly, it allows me to see what people think about the competition, which is invaluable. Since gambling is an industry with extremely tough competition, I want to use up all of the aces up my sleeve.” says Andrew Martins, senior associate at LVBet
Possible challenges for sentiment analysis
Since language contains many phrases where the syntax is similar, but the meaning is entirely different, sentiment analysis becomes challenging for the professionals behind the algorithms. Words used in a particular way can often mean something entirely different due to our use of irony, specific negations and ambiguous phrases.
Sarcasm
Something hard to detect even by humans, sarcasm poses a considerable challenge for sentiment analysis software. Most of the time, apparent clues are signifying that the sentiment is either positive or negative, while, in reality, the meaning is entirely different.
Example: I love it when buses are late!
The word love wrongly indicates that the sentiment is positive.
Context
At times, particular phrases are required to be used in context to be unambiguous. For instance, Twitter entries rarely have the necessary context to make the distinction between meanings.
Example: I hate football. -> does the person hate American football or soccer?
Metaphors
People are keen on using metaphors to express their thoughts unconventionally. Because the meaning is not literal, this creates another issue for NLP to solve.
Example: This guy is a chicken! -> we know that the guy is not a chicken, only that he’s afraid to do something. The word ‘chicken’ can be used in a positive, negative, or neutral context, hence the confusion.
Negation
Particular types of negations can confuse the algorithm at times.
Example: I don’t dislike spammers. -> this sentence is especially hard, as there’s the double negative, and the word spammers are often perceived to be negative. In reality, the sentiment is neutral here, but the algorithm might think it’s negative.
Negative terms in a positive sense
Certain negative statements become positive, as they’re a matter of perspective.
Example: This horror movie was terrifying! ->, we could assume that the sentiment is positive here, as horror movies are supposed to be scary. Still, scary is a word with a mostly negative view.
Benefits of using sentiment analysis
Sentiment analysis helps with many things connected to brand reputation management, sales, customer service, and suggestions. Here are some of its uses:
Feedback
A lot of companies receive countless comments about their products, particularly within the eCommerce industry. As there’s so much data to analyse, getting a grip on whether the customer feedback is positive or negative becomes tough to do manually. Sentiment analysis can quickly help with that.
PR disasters
Sudden drops in the sentiment of a particular phrase or brand name can indicate that there’s a crisis. Since the data is mostly provided close to real-time, reacting to the issues becomes much quicker and more effective. The faster you extinguish the fire, the less damage it causes.
“While I’ve never had any major PR crises, I managed to stop a few smaller ones in the blink of an eye. Thanks to social listening and sentiment analysis, I was able to react quickly and provide explanations, as well as any compensation if needed. Getting alerted of a sudden drop in positive sentiment and reacting to it has saved my reputation a couple of times.” remarks Dima Suponau, Founder of Number For Live Person
Upselling
By looking at which products have the best sentiment, you can upsell much more effectively. On top of this, sentiment analysis enables you to target the customers that are the most likely to respond positively to a given product or service.
Chatbot machine learning
Using sentiment analysis in chatbot technology is becoming increasingly popular among companies. Correct detection of the client’s state or emotions makes communication more effective.
Politics
Political sentiment analysis can indicate whether people respond to a campaign positively or negatively. Twitter is a precious source for such information. This, in turn, can lead to useful insights for the campaign organisers and allows them to make the appropriate adjustments.
Competitor analysis
By keeping track of your competitors’ social media efforts, you can see what works and what doesn’t. On top of this, you get to see the areas that need improvement and can, therefore, react appropriately with your own business.
“Analysing my competitors’ marketing actions has been the primary source for inspiration when it comes to my campaigns. Each time I enter a new market with a project, I check out what my competition is doing. Sentiment analysis has been beneficial with that.” says Neal Taparia, Founder of Solitaired
Use cases
To illustrate how powerful brands and companies use sentiment analysis to their advantage, here are some successful use cases:
Twitter, IBM, and Intel
Seeing that there are a lot of upsides to using sentiment analysis, the top corporations make use of it, too. For example, Twitter, IBM, and Intel use sentiment analysis to detect any employee concerns. They can then prepare specific plans to convince the employees to keep working for them based on that information.
Uber
As a rapidly-growing company, Uber had to stay on top of the sentiment people have for their brand. Because the customer’s opinion matters the most, Uber devised its own system for monitoring the sentiment. The company would analyse the brand mentions from all over the internet to identify areas in which they need to make fixes to retain their trust in them. The system was successful, and Uber still uses it day-to-day to stay on top.
Queen’s University
Canada’s Queen’s University posted a study where their researchers used sentiment analysis to predict stock market trends. By monitoring news articles, Wikipedia entries, social media, and the analysis of moneycontrol.com, they managed to find a pattern.
“Using only news sentiments, we achieved a directional accuracy of 70.59% in predicting the trends in short-term stock price movement.”
Obama’s administration
In 2012, Obama’s administration used social media sentiment analysis for measuring their campaign’s success. The findings indicated that Obama was miles ahead of Mitt Romney and tended to exhibit a much more positive sentiment to the former. If any issues popped up, Obama’s campaign team could immediately address them.
Frankfurt Stock Exchange
The Frankfurt Stock Exchange posts a weekly analysis of the stock market expectations. This provides invaluable insights to the stockbrokers, encouraging them to invest more. By surveying their investors, they’re able to analyse the possible future trends of the stock market.
Best tools for sentiment analysis
Now that you know the upsides to use sentiment analysis, here are some of the best providers:
Brand24
Founded in 2010 by Michał Sadowski, Brand24 is a Polish suite of tools that can provide you with all the information you need to manage your reputation with social listening and media monitoring successfully. Having an established position in the SaaS market, Brand24 is used by big players such as Carlsberg, H&M and Intel. It analyses the data in real-time, giving you invaluable insights in terms of your brand’s health. Brand24 uses all the leading technologies, including machine learning and natural language processing, to provide you with the most accurate data possible.
I like Brand24 the most because it has a simple interface, works beautifully, and is relatively cheap.
The pricing starts at $49, but you can try the tool out with Brand 24’s 14-day free trial.
Brandwatch
Established back in 2007, Brandwatch is the most significant player in the media monitoring market. This Brighton-based platform has a lot going for it with well-developed AI, instant reporting, an enormous database, and precise analytics. Some of the largest corporations in the world choose to use Brandwatch for its brand reputation management efforts. These include Unilever, Nestle, and Sky.
Unfortunately, Brandwatch doesn’t list its pricing options on its website, but you can book a meeting with them.
Mention
Mention is the largest platform in the industry. Boasting over nine years of experience, Mention has been in a state of constant growth ever since its launch and serves big names such as BenQ, Spendesk, and Edelman. With its database of over one billion sources, social media publishing features and AI-based insights, Mention is the ultimate social media platform.
The pricing starts at $25 a month, although this package is relatively limited, with only two keywords to monitor.
Wrap-up
Sentiment analysis has a broad spectrum of users across several industries. It provides countless insights for thousands of businesses worldwide. It became so much more than just a buzzword—it’s a technology put to use by the world’s most successful companies. Tapping into the enormous amount of data we have nowadays can give you a different understanding of the market, your brand and the competition.
Since modern companies are becoming more and more conscious of the power of sentiment analysis, this is the perfect time to get interested in it and stay one step ahead of the competitors.
Author Bio: Jan Suski is a content manager at chillifruit.com web consulting boutique agency based in London.